Exercise VII: Decision Trees and Random Forests#
Decision trees are as easy to implement with sklearn
as any other of the models we’ve studied so far. As a quick example we could try to classify the iris dataset which we’re already familiar with:
Show code cell source
import warnings
import pandas as pd
from sklearn.datasets import load_iris
from sklearn.model_selection import train_test_split
from sklearn.tree import DecisionTreeClassifier, plot_tree
warnings.filterwarnings("ignore")
data = load_iris(as_frame=True)
X, y = data.data, data.target
y = y.replace({index: name for index, name in enumerate(data["target_names"])})
X_train, X_test, y_train, y_test = train_test_split(X, y, test_size=0.2, random_state=42)
decision_tree = DecisionTreeClassifier(random_state=42)
_ = decision_tree.fit(X_train, y_train)
Trees are even easy to visualize, thanks to the plot_tree()
function:
import matplotlib.pyplot as plt
fig, ax = plt.subplots(figsize=(16, 12))
_ = plot_tree(decision_tree,
class_names=data["target_names"].tolist(),
feature_names=data["feature_names"],
filled=True,
fontsize=11,
node_ids=True,
proportion=True,
rounded=True,
ax=ax)
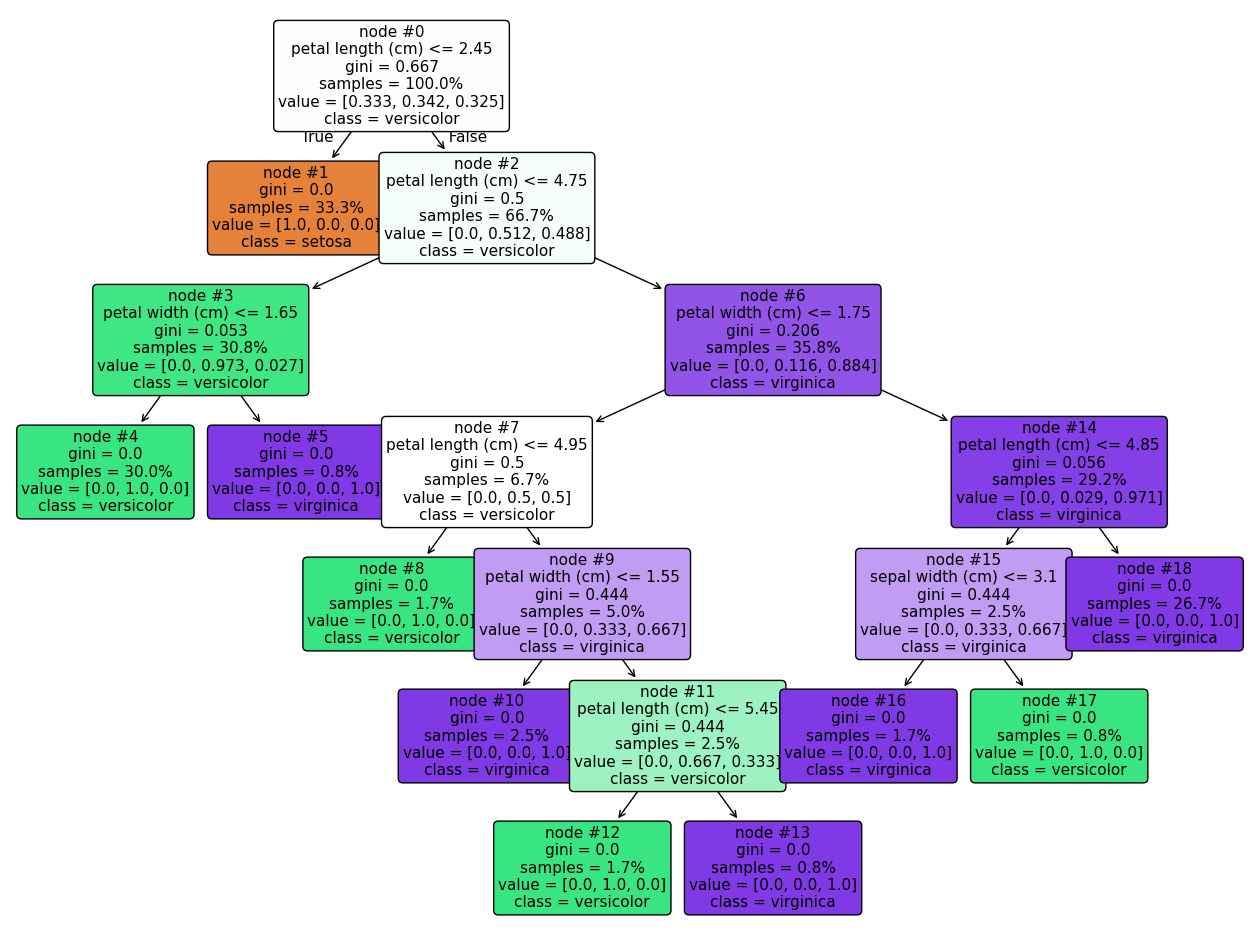
Finally, we can evaluate the model’s performance using the appropriate metrics, e.g.:
from sklearn.metrics import ConfusionMatrixDisplay
disp = ConfusionMatrixDisplay.from_estimator(decision_tree,
X_test,
y_test,
cmap=plt.cm.Blues,
normalize="true",
display_labels=data["target_names"])
disp.ax_.set_title("Confusion Matrix")
Text(0.5, 1.0, 'Confusion Matrix')
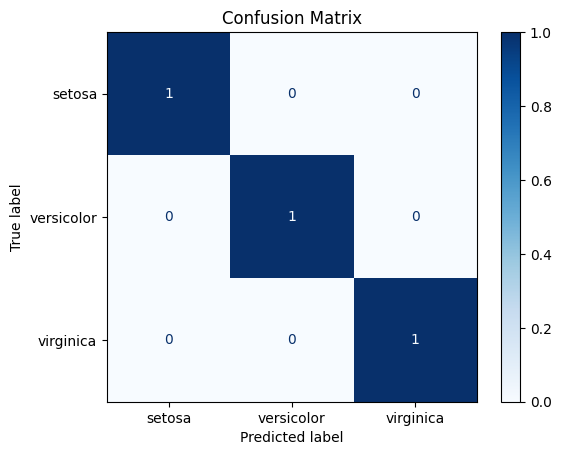
from sklearn.metrics import classification_report
y_predicted = decision_tree.predict(X_test)
print(classification_report(y_test, y_predicted))
precision recall f1-score support
setosa 1.00 1.00 1.00 10
versicolor 1.00 1.00 1.00 9
virginica 1.00 1.00 1.00 11
accuracy 1.00 30
macro avg 1.00 1.00 1.00 30
weighted avg 1.00 1.00 1.00 30
Predicting ASD Diagnosis:#
Again, we will try and create a model to classify ASD diagnosis using four FreeSurfer metrics extracted over 360 brain regions. In the previous exercise we used an \(\ell_2\) regularized logistic regression model (estimated using the LogisticRegressionCV
class) to select and fit a model, this time we will use the RandomForestClassifier
and GradientBoostingClassifier
to do the same.
Show code cell source
from sklearn.model_selection import train_test_split
from sklearn.preprocessing import StandardScaler
# Load
tsv_url = "https://raw.githubusercontent.com/neurohackademy/nh2020-curriculum/master/tu-machine-learning-yarkoni/data/abide2.tsv"
data = pd.read_csv(tsv_url, delimiter="\t")
# Clean
IGNORED_COLUMNS = ["age_resid", "sex"]
REPLACE_DICT = {"group": {1: "ASD", 2: "Control"}}
data.drop(columns=IGNORED_COLUMNS, inplace=True)
data.replace(REPLACE_DICT, inplace=True)
# Feature matrix
X = data.filter(regex="^fs").copy() # Select columns starting with "fs"
scaler = StandardScaler()
X.loc[:, :] = scaler.fit_transform(X.loc[:, :])
# Target vector
y = data["group"] == "ASD"
# Train/test split
TRAIN_SIZE = 900
X_train, X_test, y_train, y_test = train_test_split(X,
y,
train_size=TRAIN_SIZE,
random_state=0)
Random Forest#
Model Creation#
from sklearn.ensemble import RandomForestClassifier
random_forest = RandomForestClassifier(random_state=0)
_ = random_forest.fit(X_train, y_train)
Model Application#
y_predicted = random_forest.predict(X_test)
Model Evaluation#
Confusion Matrix#
disp = ConfusionMatrixDisplay.from_estimator(random_forest,
X_test,
y_test,
cmap=plt.cm.Blues,
normalize="true")
disp.ax_.set_title("Confusion Matrix")
Text(0.5, 1.0, 'Confusion Matrix')
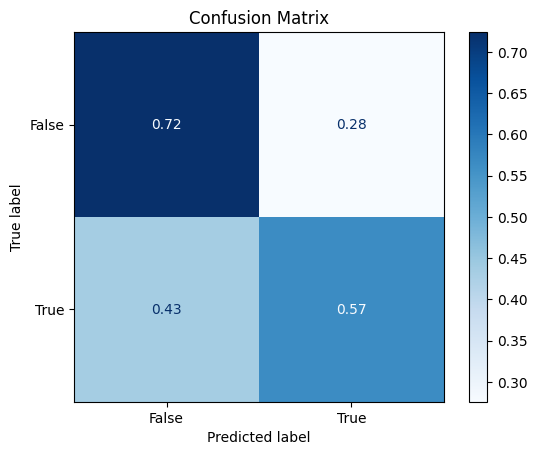
Classification Report#
print(classification_report(y_test, y_predicted))
precision recall f1-score support
False 0.68 0.72 0.70 58
True 0.62 0.57 0.59 46
accuracy 0.65 104
macro avg 0.65 0.64 0.65 104
weighted avg 0.65 0.65 0.65 104
Not too bad! We’ve improved our accuracy from 0.63 to 0.65.
Feature Importance#
One of the greatest things about trees is their interpretability. sklearn
exposes one measure (“Gini importance”) as a built-in property of the fitted random forest estimator:
random_forest.feature_importances_
array([2.96342846e-04, 5.08910846e-05, 6.69469236e-04, ...,
5.30759983e-04, 2.16033616e-04, 4.88856474e-04], shape=(1440,))
However, the documentation states that:
“impurity-based feature importances can be misleading for high cardinality features (many unique values).”
In our case, having numerical features, we would be better off using the permutation_importance()
function (for more information, see Permutation Importance vs Random Forest Feature Importance).
from sklearn.inspection import permutation_importance
importance = permutation_importance(random_forest,
X_test,
y_test,
random_state=0,
n_jobs=8)
---------------------------------------------------------------------------
KeyboardInterrupt Traceback (most recent call last)
Cell In[12], line 3
1 from sklearn.inspection import permutation_importance
----> 3 importance = permutation_importance(random_forest,
4 X_test,
5 y_test,
6 random_state=0,
7 n_jobs=8)
File /opt/hostedtoolcache/Python/3.11.11/x64/lib/python3.11/site-packages/sklearn/utils/_param_validation.py:216, in validate_params.<locals>.decorator.<locals>.wrapper(*args, **kwargs)
210 try:
211 with config_context(
212 skip_parameter_validation=(
213 prefer_skip_nested_validation or global_skip_validation
214 )
215 ):
--> 216 return func(*args, **kwargs)
217 except InvalidParameterError as e:
218 # When the function is just a wrapper around an estimator, we allow
219 # the function to delegate validation to the estimator, but we replace
220 # the name of the estimator by the name of the function in the error
221 # message to avoid confusion.
222 msg = re.sub(
223 r"parameter of \w+ must be",
224 f"parameter of {func.__qualname__} must be",
225 str(e),
226 )
File /opt/hostedtoolcache/Python/3.11.11/x64/lib/python3.11/site-packages/sklearn/inspection/_permutation_importance.py:287, in permutation_importance(estimator, X, y, scoring, n_repeats, n_jobs, random_state, sample_weight, max_samples)
284 scorer = check_scoring(estimator, scoring=scoring)
285 baseline_score = _weights_scorer(scorer, estimator, X, y, sample_weight)
--> 287 scores = Parallel(n_jobs=n_jobs)(
288 delayed(_calculate_permutation_scores)(
289 estimator,
290 X,
291 y,
292 sample_weight,
293 col_idx,
294 random_seed,
295 n_repeats,
296 scorer,
297 max_samples,
298 )
299 for col_idx in range(X.shape[1])
300 )
302 if isinstance(baseline_score, dict):
303 return {
304 name: _create_importances_bunch(
305 baseline_score[name],
(...)
309 for name in baseline_score
310 }
File /opt/hostedtoolcache/Python/3.11.11/x64/lib/python3.11/site-packages/sklearn/utils/parallel.py:77, in Parallel.__call__(self, iterable)
72 config = get_config()
73 iterable_with_config = (
74 (_with_config(delayed_func, config), args, kwargs)
75 for delayed_func, args, kwargs in iterable
76 )
---> 77 return super().__call__(iterable_with_config)
File /opt/hostedtoolcache/Python/3.11.11/x64/lib/python3.11/site-packages/joblib/parallel.py:2007, in Parallel.__call__(self, iterable)
2001 # The first item from the output is blank, but it makes the interpreter
2002 # progress until it enters the Try/Except block of the generator and
2003 # reaches the first `yield` statement. This starts the asynchronous
2004 # dispatch of the tasks to the workers.
2005 next(output)
-> 2007 return output if self.return_generator else list(output)
File /opt/hostedtoolcache/Python/3.11.11/x64/lib/python3.11/site-packages/joblib/parallel.py:1650, in Parallel._get_outputs(self, iterator, pre_dispatch)
1647 yield
1649 with self._backend.retrieval_context():
-> 1650 yield from self._retrieve()
1652 except GeneratorExit:
1653 # The generator has been garbage collected before being fully
1654 # consumed. This aborts the remaining tasks if possible and warn
1655 # the user if necessary.
1656 self._exception = True
File /opt/hostedtoolcache/Python/3.11.11/x64/lib/python3.11/site-packages/joblib/parallel.py:1762, in Parallel._retrieve(self)
1757 # If the next job is not ready for retrieval yet, we just wait for
1758 # async callbacks to progress.
1759 if ((len(self._jobs) == 0) or
1760 (self._jobs[0].get_status(
1761 timeout=self.timeout) == TASK_PENDING)):
-> 1762 time.sleep(0.01)
1763 continue
1765 # We need to be careful: the job list can be filling up as
1766 # we empty it and Python list are not thread-safe by
1767 # default hence the use of the lock
KeyboardInterrupt:
Show code cell source
import numpy as np
MEASUREMENT_DICT = {
"fsArea": "Surface Area",
"fsCT": "Cortical Thinkness",
"fsVol": "Cortical Volume",
"fsLGI": "Local Gyrification Index"
}
HEMISPHERE_DICT = {"L": "Left", "R": "Right"}
REGION_IDS = range(1, 181)
FEATURE_INDEX = pd.MultiIndex.from_product(
[HEMISPHERE_DICT.values(), REGION_IDS,
MEASUREMENT_DICT.values()],
names=["Hemisphere", "Region ID", "Measurement"])
COLUMN_NAMES = ["Identifier", "Importance"]
def parse_importance(X: pd.DataFrame, importance: np.ndarray) -> dict:
feature_info = pd.DataFrame(index=FEATURE_INDEX, columns=COLUMN_NAMES)
for i, column_name in enumerate(X_train.columns):
measurement, hemisphere, identifier, _ = column_name.split("_")
measurement = MEASUREMENT_DICT.get(measurement)
hemisphere = HEMISPHERE_DICT.get(hemisphere)
region_id = i % 180 + 1
feature_info.loc[(hemisphere, region_id,
measurement), :] = identifier, importance[i]
return feature_info
importance_series = parse_importance(X, importance["importances_mean"])
Show code cell source
import nibabel as nib
from nilearn import datasets
from nilearn import plotting
from nilearn import surface
from nilearn.image import new_img_like
HCP_NIFTI_PATH = "../chapter_06/HCP-MMP1_on_MNI152_ICBM2009a_nlin.nii.gz"
HCP_IMAGE = nib.load(HCP_NIFTI_PATH)
HCP_DATA = np.round(HCP_IMAGE.get_fdata())
def plot_importance(feature_importance: pd.DataFrame,
measurement: str) -> None:
"""
Plots coefficient estimation results using a "glass brain" plot.
Parameters
----------
coefficient_values : pd.DataFrame
Formatted dataframe containing coefficient values indexed by
(Hemisphere, Region ID, Measurement)
measurement: str
String identifier for the desired type of measurement
"""
# Create a copy of the HCP-MMP1 atlas array
template = HCP_DATA.copy()
# Replace region indices in the template with their matching importance values
for hemisphere in HEMISPHERE_DICT.values():
# Query appropriate rows
selection = feature_importance.xs((hemisphere, measurement),
level=("Hemisphere", "Measurement"))
# Extract an array of importance values
values = selection["Importance"].values
if (values > 0).any():
# Replace region indices with values
for region_id in REGION_IDS:
template_id = region_id if hemisphere == "Left" else region_id + 180
template[template == template_id] = values[region_id - 1]
else:
return
# Create a `nibabel.nifti1.Nifti1Image` instance for `plot_glass_brain`
importance_nifti = new_img_like(HCP_IMAGE, template, HCP_IMAGE.affine)
_ = plotting.plot_glass_brain(importance_nifti,
display_mode='ortho',
colorbar=True,
title=measurement)
for measurement in MEASUREMENT_DICT.values():
plot_importance(importance_series, measurement)
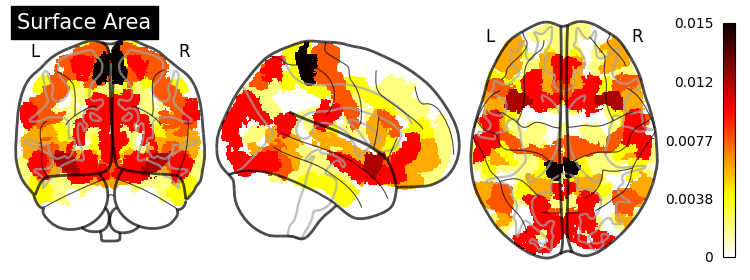
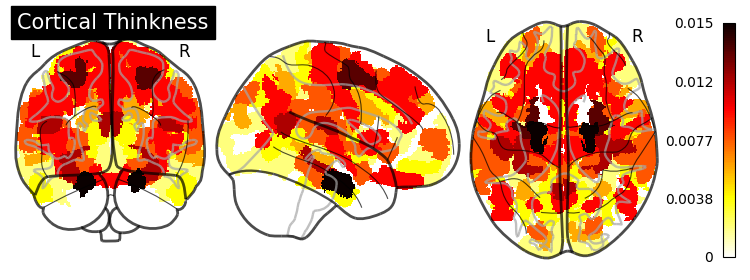
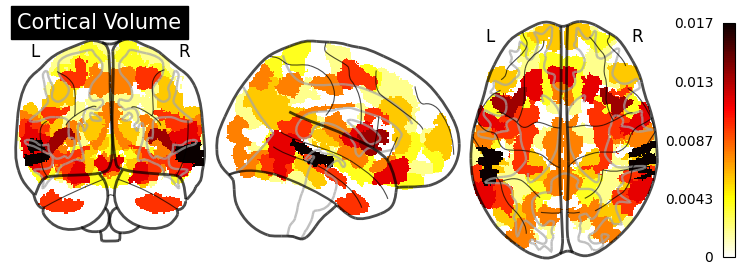
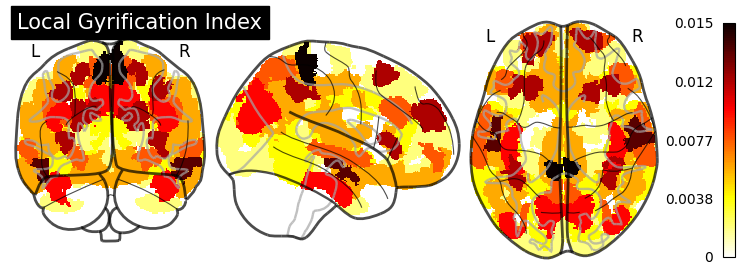
Gradient Boosting#
Model Creation#
from sklearn.ensemble import GradientBoostingClassifier
gradient_boosting = GradientBoostingClassifier(random_state=0)
_ = gradient_boosting.fit(X_train, y_train)
Model Application#
y_predicted_gb = gradient_boosting.predict(X_test)
Model Evaluation#
Confusion Matrix#
disp = ConfusionMatrixDisplay.from_estimator(gradient_boosting,
X_test,
y_test,
cmap=plt.cm.Blues,
normalize="true")
disp.ax_.set_title("Confusion Matrix")
Text(0.5, 1.0, 'Confusion Matrix')
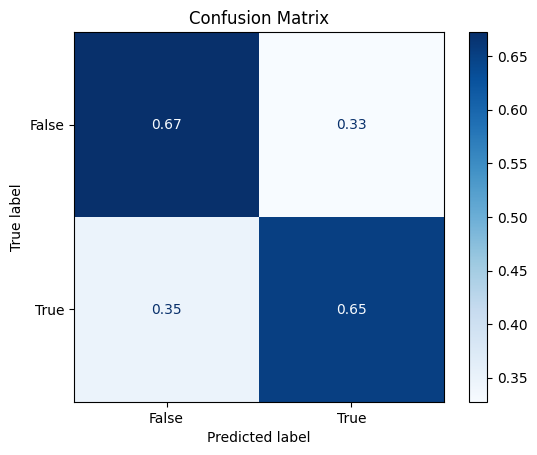
Classification Report#
print(classification_report(y_test, y_predicted_gb))
precision recall f1-score support
False 0.71 0.67 0.69 58
True 0.61 0.65 0.63 46
accuracy 0.66 104
macro avg 0.66 0.66 0.66 104
weighted avg 0.67 0.66 0.66 104
We’ve ended up with an overall slightly better accuracy and precision.
Feature Importance#
from sklearn.inspection import permutation_importance
gb_importance = permutation_importance(gradient_boosting,
X_test,
y_test,
random_state=0,
n_jobs=8)
Show code cell source
gb_importance_series = parse_importance(X, gb_importance["importances_mean"])
for measurement in MEASUREMENT_DICT.values():
plot_importance(gb_importance_series, measurement)
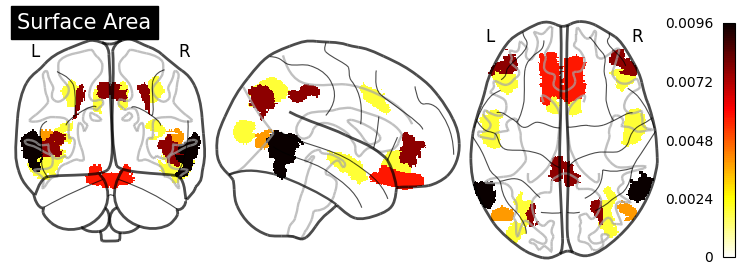
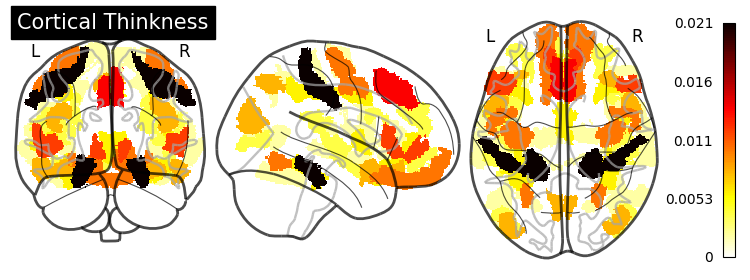
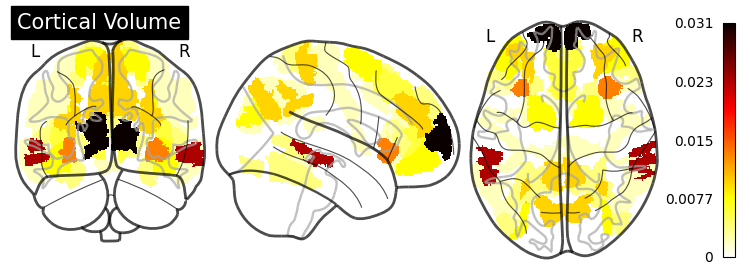
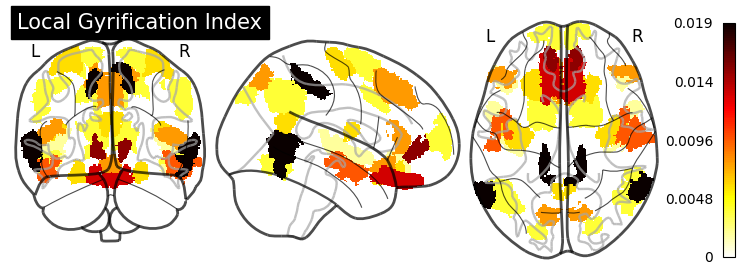
Hyperparameter Tuning#
Grid Search#
from sklearn.model_selection import GridSearchCV
N_ESTIMATORS = range(60, 130, 10)
MAX_DEPTH = range(2, 5)
PARAM_GRID = {"n_estimators": N_ESTIMATORS, "max_depth": MAX_DEPTH}
estimator = GradientBoostingClassifier(random_state=0)
model_searcher = GridSearchCV(estimator,
param_grid=PARAM_GRID,
return_train_score=True)
Model Selection#
_ = model_searcher.fit(X_train, y_train)
Selected Model Application#
y_predicted_grid = model_searcher.predict(X_test)
Selected Model Evaluation#
model_searcher.best_params_
{'max_depth': 3, 'n_estimators': 70}
model_searcher.best_score_
0.6322222222222222
Confusion Matrix#
disp = ConfusionMatrixDisplay.from_estimator(model_searcher,
X_test,
y_test,
cmap=plt.cm.Blues,
normalize="true")
disp.ax_.set_title("Confusion Matrix")
Text(0.5, 1.0, 'Confusion Matrix')
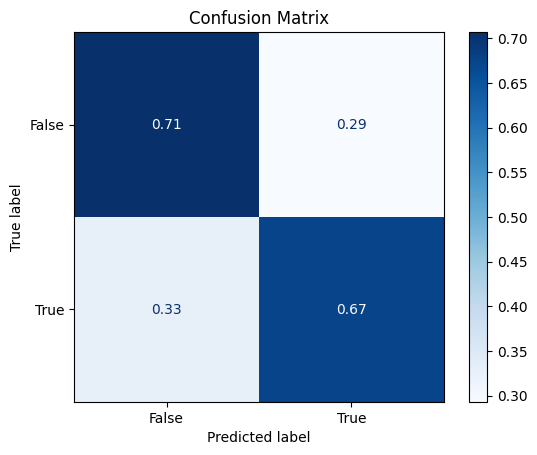
Classification Report#
print(classification_report(y_test, y_predicted_grid))
precision recall f1-score support
False 0.73 0.71 0.72 58
True 0.65 0.67 0.66 46
accuracy 0.69 104
macro avg 0.69 0.69 0.69 104
weighted avg 0.69 0.69 0.69 104
Model Selection#
COLORS = "red", "green", "blue"
PARAM_COLUMNS = [
"param_max_depth", "param_n_estimators", "mean_train_score",
"mean_test_score", "std_train_score", "std_test_score"
]
PARAM_COLUMN_NAMES = [
"Max Depth", "Number of Estimators", "Mean Train Score", "Mean Test Score",
"Train Score STD", "Test Score STD"
]
def plot_search_results(cv_results: dict):
data = pd.DataFrame(cv_results)[PARAM_COLUMNS].copy()
data.columns = PARAM_COLUMN_NAMES
param_fig, param_ax = plt.subplots(figsize=(10, 5))
param_ax.set_title(
"GridSearchCV Test-set Accuracy by Number of Estimators and Max. Depth"
)
for i, (key, grp) in enumerate(data.groupby(["Max Depth"])):
grp.plot(kind="line",
x="Number of Estimators",
y="Mean Test Score",
color=COLORS[i],
label=str(key),
ax=param_ax)
score_mean = grp["Mean Test Score"]
score_std = grp["Test Score STD"]
score_lower_limit = score_mean - score_std
score_upper_limit = score_mean + score_std
param_ax.fill_between(N_ESTIMATORS,
score_lower_limit,
score_upper_limit,
color=COLORS[i],
alpha=0.1)
param_ax.set_ylabel("Accuracy")
param_ax.scatter(model_searcher.best_params_["n_estimators"],
model_searcher.best_score_,
marker="*",
color="black",
s=150,
label="Selected Model")
_ = param_ax.legend()
plot_search_results(model_searcher.cv_results_)
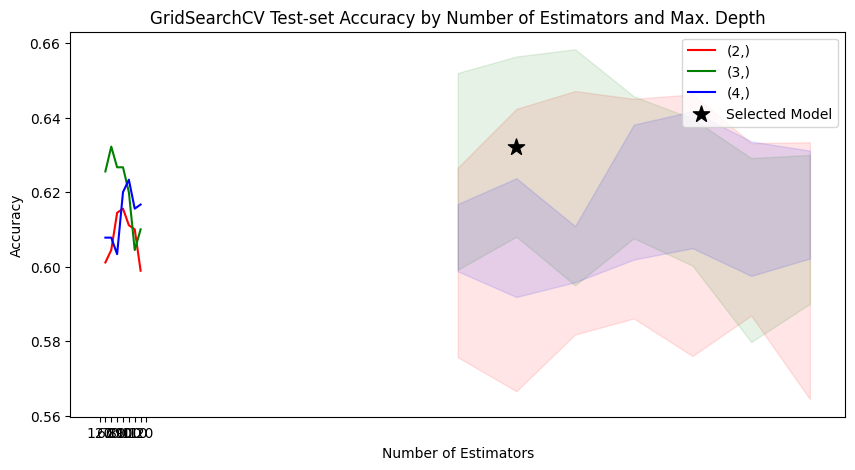
What would happen if we changed cv
from it’s default value (5) to 10? Would GridSearchCV
select the same model?
model_searcher_2 = GridSearchCV(estimator,
param_grid=PARAM_GRID,
return_train_score=True,
cv=10)
Model Selection#
_ = model_searcher_2.fit(X_train, y_train)
Selected Model Application#
y_predicted_grid_2 = model_searcher_2.predict(X_test)
Selected Model Evaluation#
model_searcher_2.best_params_
{'max_depth': 3, 'n_estimators': 120}
model_searcher_2.best_score_
0.6199999999999999
Confusion Matrix#
disp = ConfusionMatrixDisplay.from_estimator(model_searcher_2,
X_test,
y_test,
cmap=plt.cm.Blues,
normalize="true")
disp.ax_.set_title("Confusion Matrix")
Text(0.5, 1.0, 'Confusion Matrix')
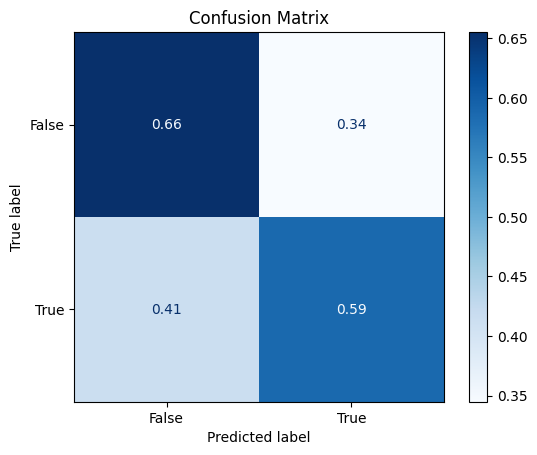
Classification Report#
print(classification_report(y_test, y_predicted_grid_2))
precision recall f1-score support
False 0.67 0.66 0.66 58
True 0.57 0.59 0.58 46
accuracy 0.62 104
macro avg 0.62 0.62 0.62 104
weighted avg 0.63 0.62 0.63 104
Feature Importance#
grid_importance = permutation_importance(model_searcher_2,
X_test,
y_test,
random_state=0,
n_jobs=8)
Show code cell source
grid_importance_series = parse_importance(X,
grid_importance["importances_mean"])
for measurement in MEASUREMENT_DICT.values():
plot_importance(grid_importance_series, measurement)
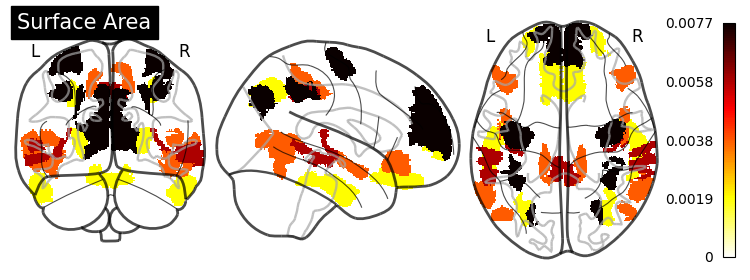
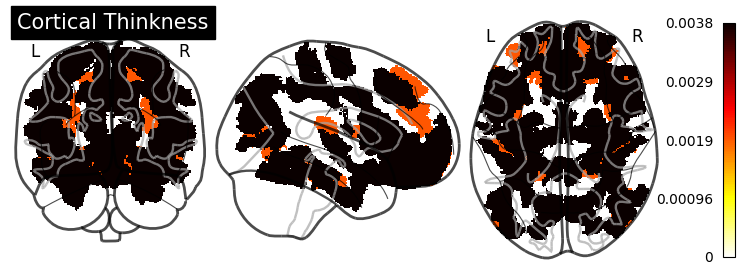
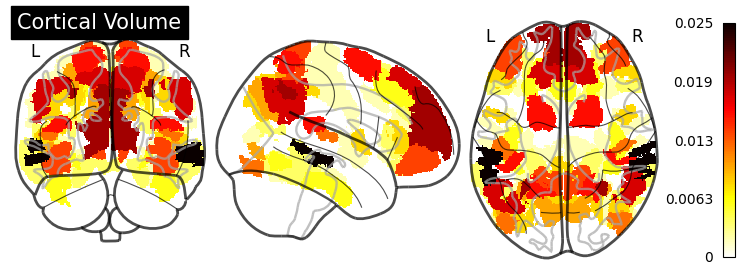
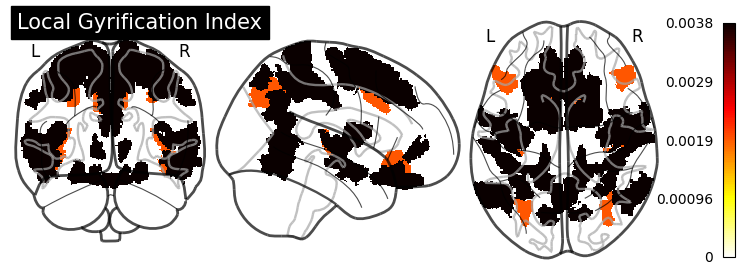
SHAP (SHapley Additive exPlanations) values show how each feature affects each final prediction, the significance of each feature compared to others, and the model’s reliance on the interaction between features:
import shap
shap_values = shap.TreeExplainer(model_searcher_2.best_estimator_).shap_values(X_train)
shap.summary_plot(shap_values, X_train, plot_type="bar", max_display=10)
shap.summary_plot(shap_values, X_train, max_display=10)
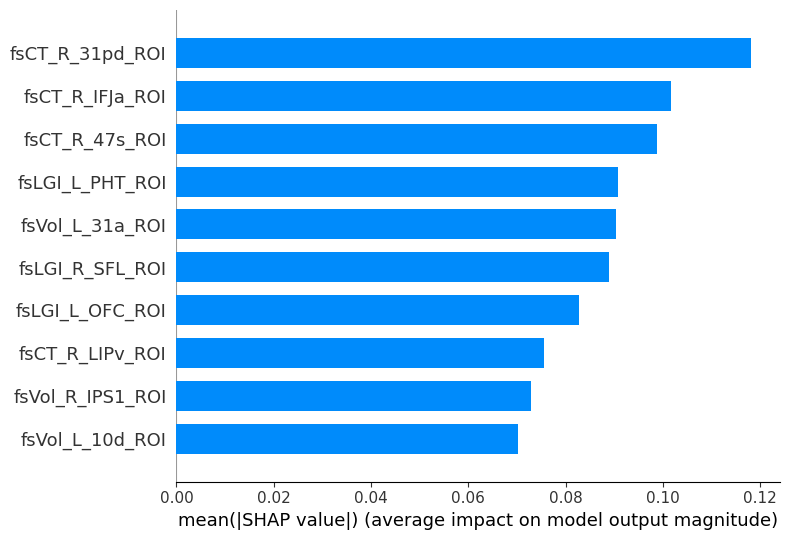
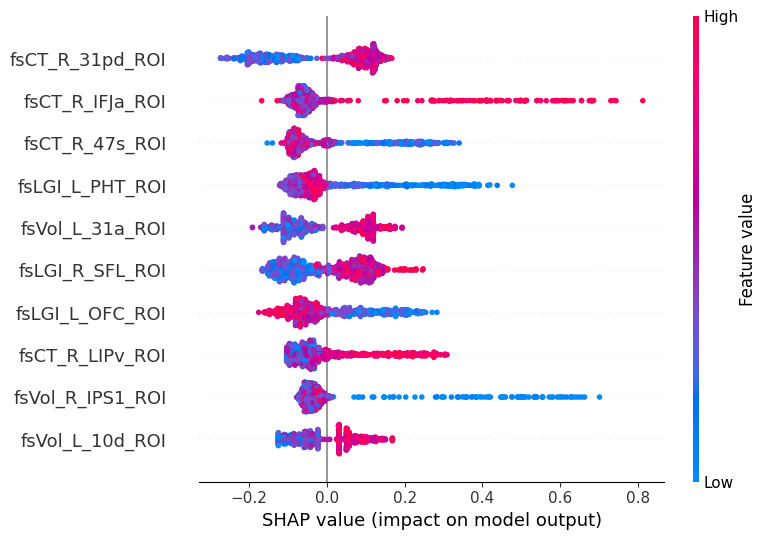